Artificial intelligence (AI) can provide computers with the ability to learn and replicate human intelligence, and have intentionality, intelligence, and adaptability as core qualities. Machine learning (ML) focuses on creating programmes that can make predictions to help the decision-making process and is used widely in optimizing search engines, e-commerce sites.
In AI, the goal is to create a computer system that mimics the human brain to solve complex problems. In the case of ML, the objective is to create a computer system that can learn from data to explore trends, patterns and predict an outcome. AI helps the computer or the machine think, which means that it will make its own decision without any human intervention.
ML can be described as a subset of AI. It provides the statistical tools to explore and understand a particular set of data. ML can find patterns in the data and apply those patterns to solve futuristic problems. ML can be further classified into different approaches:
- Supervised machine learning,
- Unsupervised machine learning, and
- Reinforcement learning or semi-supervised machine learning
Supervised Machine Learning: In supervised machine learning, a supervised machine learning model can be created. Supervised machine learning uses labeled data to build algorithms to classify data or predict outcomes. As part of the model building process, input data is provided; it adjusts its weights until fitted appropriately. Supervised learning can solve complex real-world problems, and its examples include spam detection. In spam detection, institutions build models that look for patterns or anomalies in new data to organize spam and non-spam-related content.
Unsupervised Machine Learning: Unsupervised learning or unsupervised machine learning employs models that are not supervised; instead, models find the patterns from the given data. Unsupervised machine learning can be compared to learning akin to the human brain learning new things. Unsupervised machine learning deals with situations in machine learning models to find relevant patterns. Examples of unsupervised machine learning include customer segmentation or content recommendation.
Semi-supervised machine learning: Semi-supervised machine learning utilizes attributes of both supervised and unsupervised machine learning. It employs a small amount of labeled data and a large amount of unlabeled data to reap the benefits of both unsupervised and supervised learning, which means the model can be trained to label data without using as much labeled training data. A text document classifier is a pretty common example of semi-supervised machine learning.
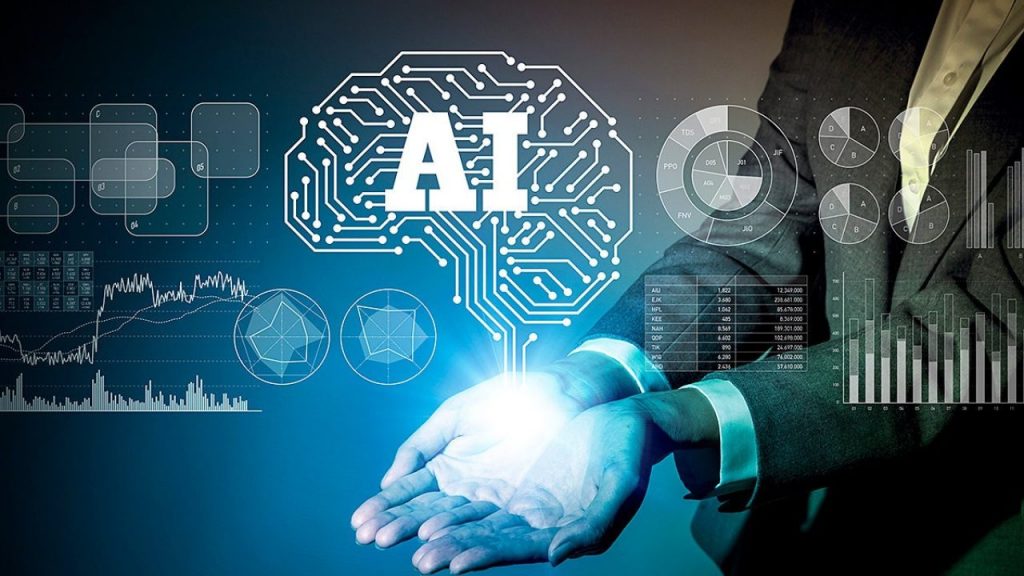
There have been several initiatives to catalyze the application of AI and ML in the social sector. The social sector initiative like UN Global Pulse Labs and corporate programmes, such as Google AI for Good Grants, Microsoft AI for Humanity, Mastercard Center for Inclusive Growth, and the Rockefeller Foundation’s Data Science for Social Impact have been critical in building the ecosystem.
Though AI and ML are still nascent compared to their application in the commercial sector, it has started to gain traction. It can be critical in improving efficiency in addressing the Sustainable Development Goals (SDGs). Because the social sector generates enormous amounts of data, successful and sustainable integration of AI and machine learning to benefit the SDGs can be ensured only by guaranteeing security and transparency in handling Big Data. It is necessary to build trust in the machines that will be helping humans sustainably do their excellent work, as demanded by the SDGs. AI applications should maximize a net positive effect on as many SDGs as possible. Therefore, there should be good social applications across the five areas of people, planet, prosperity, peace, and partnerships, the targets of the sustainable development agenda.
Kultar Singh – Chief Executive Officer, Sambodhi